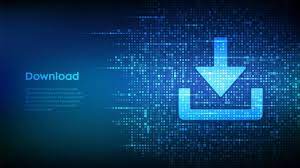

4: Use data profiling early and oftenĭata quality profiling is the process of examining data from an existing source and summarizing information about the data. An enterprise wide DQ standard will help educate all involved parties and make the adoption seamless. “This will enable stakeholders across the enterprise to understand and execute their business operations in accordance with the defined and agreed-to DQ standard,” says Chien. It is likely that different stakeholders in an enterprise will have different levels of business sensitivity, culture and maturity, so the manner and speed with which requirements of DQ enablements are met may differ. 3: Establish a DQ standard across the organizationĭ&A leaders need to establish data quality standards that can be followed across all business units in the organization. Different lines of business using the same data, for example, customer master data, may have different standards and therefore different expectations for the data quality improvement program. Data and analytics (D&A) leaders need to have periodic discussions with business stakeholders to capture their expectations. This responsibility of describing what can be defined as “good” lies with the business. To improve data quality, first it is important to understand what is “best fit” for the organization.
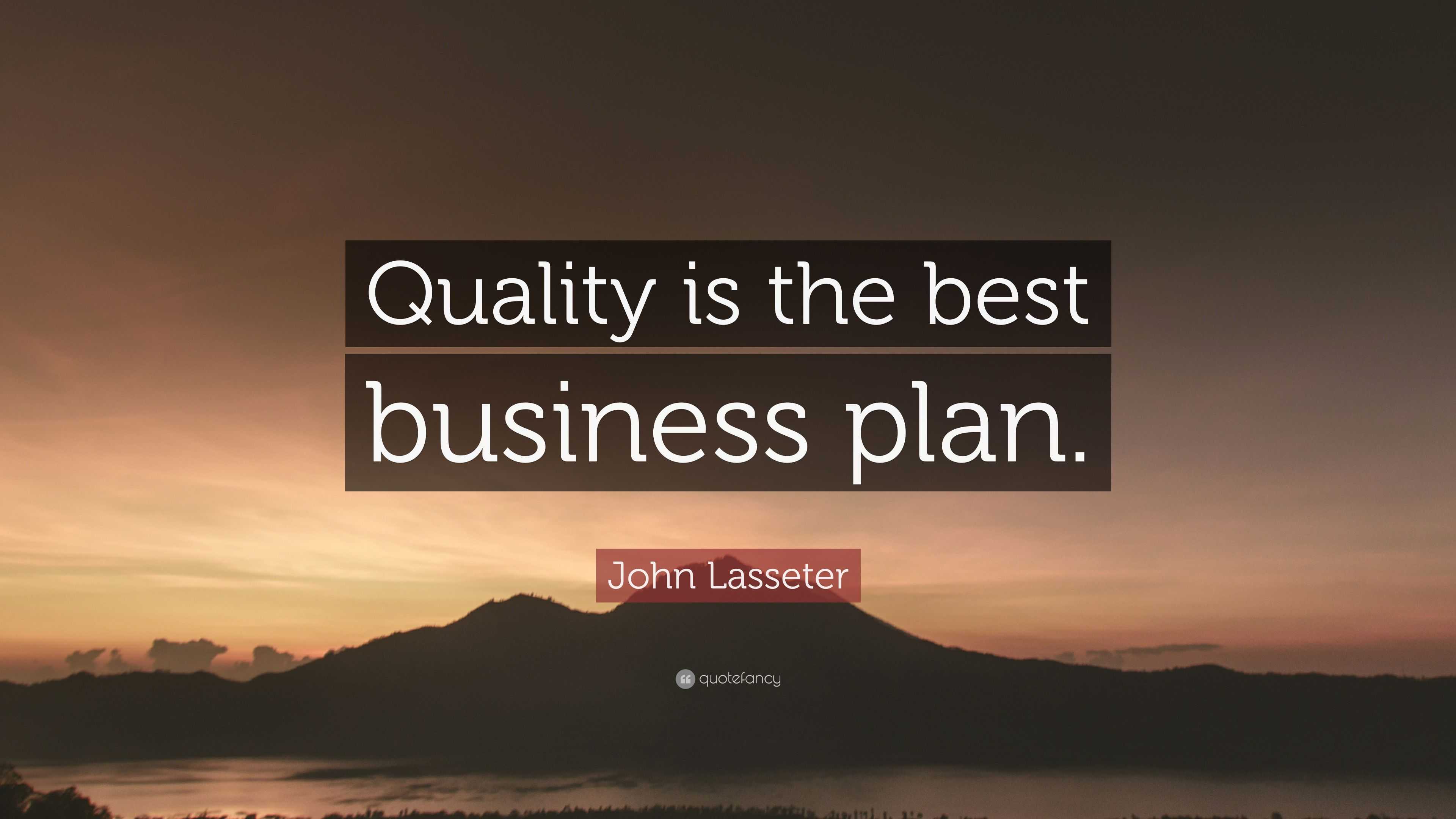
2: Define what is a “good enough” standard of data After establishing a clear connection between data as an asset and the improvement requirements, data and analytics leaders can begin building a targeted data quality improvement program that clearly defines the scope, the list of stakeholders and a high-level investment plan. Make a list of the existing data quality issues the organization is facing and how they are impacting revenue and other business KPIs. Identify a clear linkage between business processes, key performance indicators (KPIs) and data assets. 1: Establish how improved data quality impacts business decisions

Data quality is a competitive advantage that D&A leaders need to improve upon continuously.” “Good quality data provides better leads, better understanding of customers and better customer relationships. “Data quality is directly linked to the quality of decision making,” says Melody Chien, Senior Director Analyst, Gartner. Gartner predicts that by 2022, 70% of organizations will rigorously track data quality levels via metrics, improving it by 60% to significantly reduce operational risks and costs. The emphasis on data quality (DQ) in enterprise systems has increased as organizations increasingly use data analytics to help drive business decisions. Apart from the immediate impact on revenue, over the long term, poor quality data increases the complexity of data ecosystems and leads to poor decision making.ĭownload roadmap: Data Governance Best Practices Every year, poor data quality costs organizations an average $12.9 million.
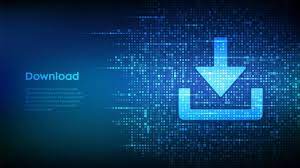